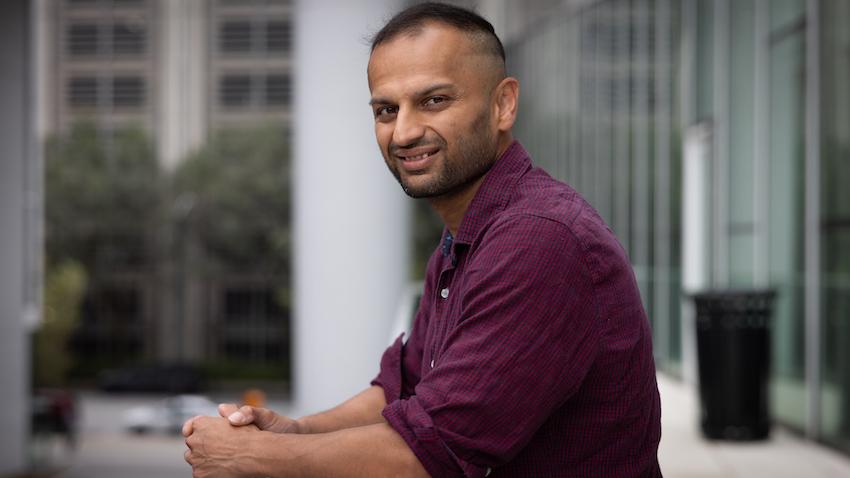
Assistant Professor Explores Analytical and Practical Benefits of Statistical NLP
Machine learning can reveal new insights about the languages of ancient texts. It can also help researchers understand the connection between these texts, their translations, and more recent texts written by authors who lived hundreds of years apart.
Kartik Goyal believes that statistical natural language processing (NLP) is the key to finding the answers.
Goyal is starting his first semester as an assistant professor in the School of Interactive Computing at Georgia Tech. He joins Georgia Tech after spending two years as a research assistant professor at the Toyota Technical Institute at Chicago. He earned his Ph.D. from Carnegie Mellon’s School of Computer Science and worked within CMU’s Language Technologies Institute.
“I’m interested in building statistical and machine learning tools that aim at capturing hidden but systematic structured information in data that occurs through human processes like language, music, and other things related to society,” Goyal said.
While there are many ways to apply NLP, Goyal advocates for a statistical approach. Statistical NLP manipulates natural languages to teach machines to read, understand, and derive meaning from human languages. The approach has analytical and practical benefits, Goyal said.
“On the one hand, we can analyze how different human languages interact with each other, and on the other hand, we can build machine translation tools that can help with communication across multiple cultures,” he said.
As a Ph.D. student, Goyal authored a paper that presented a generative model that could analyze glyph shapes in early modern books, such as Leviathan by Thomas Hobbes, published in 1651.
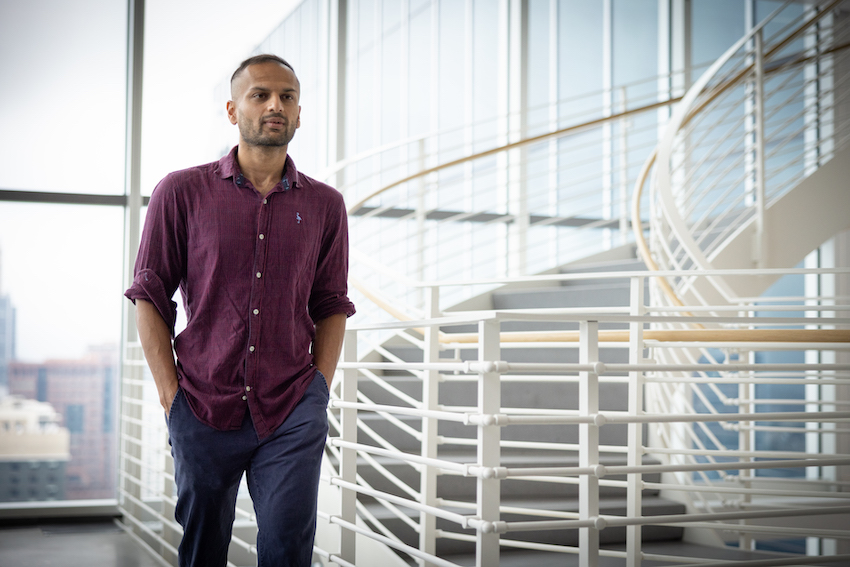
What interests you about working at Georgia Tech?
Georgia Tech attracts a lot of brilliant minds in computing, and there’s a lot of intellectual diversity. As a result, it attracts many bright students as well, so I’m looking forward to collaborating with so many people at Georgia Tech.
What will your research at Georgia Tech consist of?
I’ll continue to build on the research I started during my Ph.D. My research mainly focused on sequence models for NLP and the statistical properties of algorithms we often use for machine translation, summarization, and named entity recognition. I developed algorithms that critiqued and made improvements to existing techniques to make NLP systems better. I also developed generative models for structure-ladened variables.
What inspired you to pursue this field of research?
I came from a computer science and physics background. I think my internships had a role to play in awakening my interest in machine learning and natural language. I started out right at the intersection of machine learning and NLP in grad school. I discovered a lot of new problems, and I found these human processes, the digital humanities, fascinating.
What do you hope to accomplish in your research?
I hope to build a new lab so my collaborators, my students, and I can develop better statistical tools to aid the analysis and processing of natural language. I hope to gain insight into how these systems, like language, and music evolve naturally.
What are you looking forward to about teaching your students, and how do you plan on working with them?
I’m looking forward to teaching the graduate-level NLP course in the spring. I plan to cover many things that have become the basics for a lot of NLP researchers today. I want to bring a statistical and technical flavor to some of these topics so that students attracted to statically analyzing NLP problems can do so.
We are thrilled to announce Vivek Sarkar as the new Dean of the College of Computing at Georgia Tech! With a distinguished career spanning academia and industry, Sarkar's leadership promises to elevate our community to new heights. https://t.co/2mX5D46cJz pic.twitter.com/LxpLTCXWZV
— Georgia Tech Computing (@gtcomputing) April 12, 2024
@GeorgiaTech's dedication to excellence in computer science (CS) has been recognized once again, with the latest U.S. News and World Report rankings unveiling the institution at 7th place overall for graduate CS studies.https://t.co/qavNUSTb7n pic.twitter.com/BcGyGBQld8
— Georgia Tech Computing (@gtcomputing) April 10, 2024