Informative Features for Model Comparison.
Wittawat Jitkrittum,
Heishiro Kanagawa,
Patsorn Sangkloy,
James Hays,
Bernhard Schölkopf, and
Arthur Gretton.
NeurIPS 2018.
Paper (arXiv)
|
SwapNet: Garment Transfer in Single View Images
Amit Raj,
Patsorn Sangkloy,
Huiwen Chang,
James Hays,
Duygu Ceylan,
and Jingwan Lu.
ECCV 2018.
Project page,
Paper
|
MapNet: Geometry-Aware Learning of Maps for Camera Localization
Samarth Brahmbhatt,
Jinwei Gu,
Kihwan Kim,
James Hays, and
Jan Kautz.
CVPR 2018.
Paper (arXiv)
|
SketchyGAN: Towards Diverse and Realistic Sketch to Image Synthesis.
Wengling Chen and James Hays.
CVPR 2018.
Paper (arXiv)
|
TextureGAN: Controlling Deep Image Synthesis with Texture Patches.
Wenqi Xian,
Patsorn Sangkloy,
Varun Agrawal,
Amit Raj,
Jingwan Lu,
Chen Fang,
Fisher Yu, and James Hays.
CVPR 2018.
Paper (arXiv)
|
On Convergence and Stability of GANs.
Naveen Kodali, Jacob Abernethy, James Hays, and Zsolt Kira.
arXiv, May 2017.
Paper (arXiv)
|
DeepNav: Learning to Navigate Large Cities.
Samarth Brahmbhatt, James Hays.
CVPR 2017.
Paper (arXiv)
|
StuffNet: Using 'Stuff' to Improve Object Detection.
Samarth Brahmbhatt, Henrik I. Christensen, James Hays.
WACV 2017.
Paper (arXiv)
|
Localizing and Orienting Street Views Using Overhead Imagery.
Nam Vo, James Hays.
ECCV 2016.
Project Page,
Paper
|
COCO Attributes: Attributes for People, Animals, and Objects.
Genevieve Patterson, James Hays.
ECCV 2016.
Paper
|

WebGazer: Scalable Webcam Eye Tracking Using User Interactions.
Alexandra Papoutsaki, Patsorn Sangkloy, James Laskey, Nediyana Daskalova, Jeff Huang, James Hays.
IJCAI 2016.
Project Page,
Paper
|

Learning to Match Aerial Images with Deep Attentive Architectures.
Hani Altwaijry, Eduard Trulls, James Hays, Pascal Fua, and Serge Belongie.
CVPR 2016.
Paper
|

Solving Small-piece Jigsaw Puzzles by Growing Consensus.
Kilho Son, Daniel Moreno, James Hays, David B. Cooper.
CVPR 2016.
Project Page,
Paper
|

Tropel: Crowdsourcing Detectors with Minimal Training.
Genevieve Patterson, Grant Van Horn, Serge Belongie, Pietro Perona, James Hays
HCOMP 2015 Best paper runner-up.
Paper
|
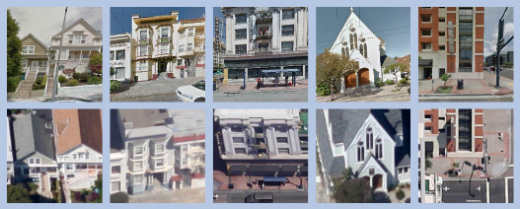
Learning Deep Representations for Ground-to-Aerial Geolocalization.
Tsung-Yi Lin, Yin Cui, Serge Belongie, and James Hays.
CVPR 2015 (Oral).
Paper
|
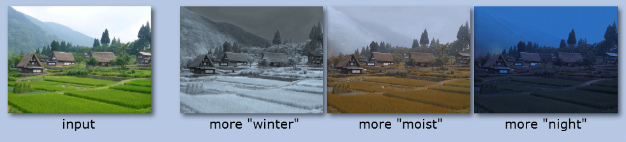
Transient Attributes for High-Level Understanding and Editing of Outdoor Scenes.
Pierre-Yves Laffont, Zhile Ren, Xiaofeng Tao, Chao Qian, and James Hays.
Siggraph 2014.
Project Page,
Paper
|
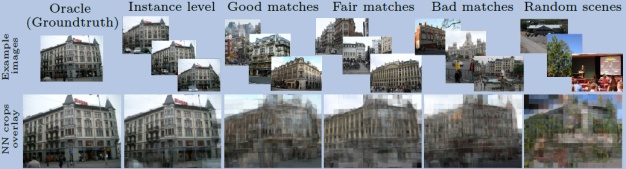
Good Image Priors for Non-blind Deconvoluton: Generic vs Specific.
Libin Sun, Sunghyun Cho, Jue Wang, and James Hays.
ECCV 2014.
Project Page
|

Solving Square Jigsaw Puzzles with Loop Constraints.
Kilho Son, James Hays, and David B. Cooper.
ECCV 2014.
Project Page, Paper
|

Microsoft COCO: Common Objects in Context.
Tsung-Yi Lin, Michael Maire, Serge Belongie, James Hays, Pietro Perona, Deva Ramanan, Piotr Dollar, and C. Lawrence Zitnick.
ECCV 2014.
Project Page,
Paper
|
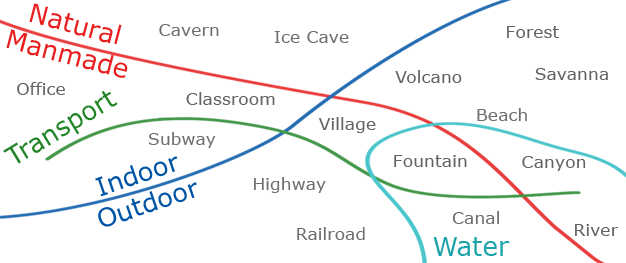
The SUN Attribute Database: Beyond Categories for Deeper Scene Understanding.
Genevieve Patterson, Chen Xu, Hang Su, and James Hays.
International Journal of Computer Vision. vol. 108:1-2, 2014. Pp 59-81.
Project Page, Paper
Previously published as:
SUN Attribute Database: Discovering, Annotating, and Recognizing Scene Attributes.
Genevieve Patterson and James Hays. CVPR 2012. Paper
|
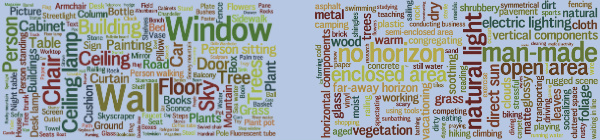
Basic level scene understanding: categories, attributes and structures.
Jianxiong Xiao, James Hays, Bryan C. Russell, Genevieve Patterson, Krista A. Ehinger, Antonio Torralba, and Aude Oliva.
Frontiers in Psychology, 2013, 4:506.
This paper is a survey of recent work related to the SUN database.
Paper
|

Cross-View Image Geolocalization.
Tsung-Yi Lin, Serge Belongie, and James Hays.
CVPR 2013.
Paper
|

FrameBreak: Dramatic Image Extrapolation by Guided Shift-Maps.
Yinda Zhang, Jianxiong Xiao, James Hays, and Ping Tan.
CVPR 2013.
Project Page,
Paper
|

Edge-based Blur Kernel Estimation Using Patch Priors.
Libin "Geoffrey" Sun,
Sunghyun Cho,
Jue Wang,
and James Hays. ICCP 2013.
Project Page,
Paper
|

Dating Historical Color Images.
Frank Palermo, James Hays, and Alexei A Efros.
ECCV 2012.
Project Page, Paper
|

How do humans sketch objects?
Mathias Eitz, James Hays, and Marc Alexa.
Transactions on Graphics (TOG) - Proceedings of ACM SIGGRAPH 2012.
Project Page, Paper
Previously presented as:
Learning to classify human object sketches
Mathias Eitz and James Hays.
ACM SIGGRAPH 2011 Talks Program.
|

Super-resolution from Internet-scale Scene Matching.
Libin "Geoffrey" Sun and James Hays.
International Conference on Computational Photography (ICCP) 2012.
Project Page, Paper
|

Quality Assessment for Crowdsourced Object Annotations.
Sirion Vittayakorn and James Hays.
British Machine Vision Conference (BMVC) 2011.
Project page, Paper, Bibtex
|

Sun database: Exploring a large collection of scene categories
Jianxiong Xiao, Krista Ehinger, James Hays, Aude Oliva, and Antonio Torralba.
International Journal of Computer Vision (IJCV) 2014.
Project page, Paper, Browse database
Previously published as:
SUN Database: Large-scale Scene Recognition from Abbey to Zoo
Jianxiong Xiao, James Hays, Krista Ehinger, Aude Oliva, and Antonio Torralba.
IEEE Conference on Computer Vision and Pattern Recognition (CVPR) 2010. Paper
Scene categorization and detection: the power of global features
James Hays, Jianxiong Xiao, Krista Ehinger, Aude Oliva, and Antonio Torralba.
Vision Sciences Society annual meeting (VSS) 2010.
We present the Scene UNderstanding (SUN) database containing 899 categories and 130,519 images. We use 397 well-sampled
categories to benchmark numerous algorithms for scene recognition. We measure human scene classification performance
on the SUN database and compare this with computational methods.
|
Ph.D. Thesis: Large Scale Scene Matching for Graphics and
Vision
Thesis Page
Our visual experience is extraordinarily varied and complex. The diversity
of the visual world makes it difficult for computer vision to understand
images and for computer graphics to synthesize visual content. But for all
its richness, it turns out that the space of "scenes" might not be
astronomically large. With access to imagery on an Internet scale,
regularities start to emerge - for most images, there exist numerous
examples of semantically and structurally similar scenes. Is it possible
to sample the space of scenes so densely that one can use similar scenes
to "brute force" otherwise difficult image understanding and manipulation
tasks? This thesis is focused on exploiting and refining large scale scene
matching to short circuit the typical computer vision and graphics
pipelines for image understanding and manipulation.
|

Image Sequence Geolocation with Human Travel Priors
Evangelos Kalogerakis, Olga Vesselova, James Hays, Alexei A. Efros, and Aaron Hertzmann.
IEEE International Conference on Computer Vision (ICCV '09)
Project Page
|
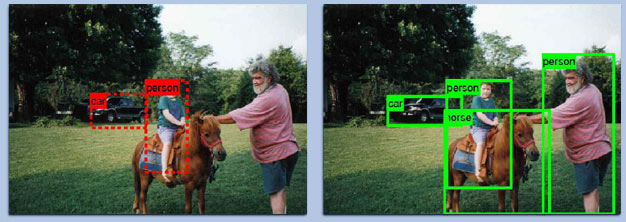
An empirical study of Context in Object Detection
Santosh Divvala, Derek Hoiem, James Hays, Alexei A. Efros, and Martial Hebert.
IEEE Conference on Computer Vision and Pattern Recognition (CVPR) 2009.
Project Page, Paper
|
New: Book chapter with expanded geolocalization experiments.
Large-Scale Image Geolocalization
James Hays and Alexei Efros.
Multimodal Location Estimation of Videos and Images. Pages 41-62. 2014.
Paper,
Bibtex
Previously published as:
IM2GPS: estimating geographic information from a single image
James Hays and Alexei Efros.
IEEE Conference on Computer Vision and Pattern Recognition (CVPR) 2008.
Paper.
CVPR 2008 Project Page. Google Tech Talk.
Abstract:
Estimating geographic information from an image is an excellent, difficult high-level computer vision problem whose
time has come. The emergence of vast amounts of geographically-calibrated image data is a great reason for computer
vision to start looking globally - on the scale of the entire planet! In this paper, we propose a simple algorithm for
estimating a distribution over geographic locations from a single image using a purely data-driven scene matching
approach. For this task, we will leverage a dataset of over 6 million GPS-tagged images from the Internet. We represent
the estimated image location as a probability distribution over the Earth's surface. We quantitatively evaluate our
approach in several geolocation tasks and demonstrate encouraging performance (up to 30 times better than chance). We
show that geolocation estimates can provide the basis for numerous other image understanding tasks such as population
density estimation, land cover estimation or urban/rural classification.
|
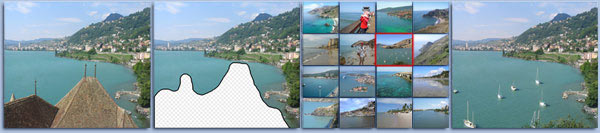
Scene Completion Using Millions of Photographs
James Hays and Alexei Efros.
Transactions on Graphics (SIGGRAPH 2007). August 2007, vol. 26, No. 3.
Project Page,
SIGGRAPH Paper,
CACM Paper,
CACM Technical Perspective by Marc Levoy,
Bibtex
Abstract: What can you do with a million images? In this paper we present a new image completion algorithm powered by a huge database of photographs gathered from the Web. The algorithm patches up holes in images by finding similar image regions in the database that are not only seamless but also semantically valid. Our chief insight is that while the space of images is effectively infinite, the space of semantically differentiable scenes is actually not that large. For many image completion tasks we are able to find similar scenes which contain image fragments that will convincingly complete the image. Our algorithm is entirely data-driven, requiring no annotations or labelling by the user. Unlike existing image completion methods, our algorithm can generate a diverse set of image completions and we allow users to select among them. We demonstrate the superiority of our algorithm over existing image completion approaches.
|
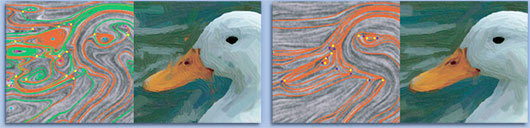
Interactive Tensor Field Design and Visualization on Surfaces
Eugene Zhang, James Hays,
and Greg Turk.
IEEE Transaction on Visualization and Computer Graphics, 2007, Vol 13(1), pp 94-107.
Project Page,
Paper, Bibtex
This research project was primarily Eugene's work and I played only a small role.
|
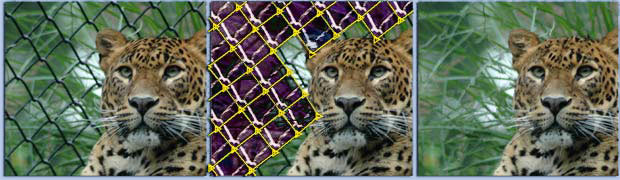
Image De-fencing
Yanxi Liu,
Tamara Belkina,
James Hays,
and Roberto Lublinerman.
IEEE Conference on Computer Vision and Pattern Recognition (CVPR) 2008.
Paper, Bibtex
We introduce a novel image segmentation algorithm that uses translational symmetry as the primary foreground/background separation cue. We use texture-based inpainting to recover an un-occluded background.
|
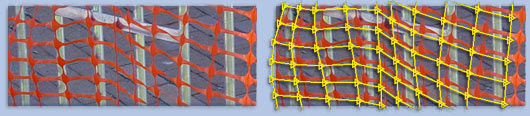
Discovering Texture Regularity as a Higher-Order Correspondence Problem
James Hays, Marius Leordeanu,
Alexei Efros,
and Yanxi Liu.
European Conference on Computer Vision (ECCV) 2006.
Paper, Bibtex
We find arbitrarily distorted regular patterns in real images by treating lattice-finding as a higher-order
assignment problem. We leverage previous work
from Marius Leordeanu and Martial Hebert to approximate the optimal assignment under second-order constraints.
Source code available upon request, although this
code by Minwoo Park et al. is likely more accurate and faster.
|

Quantitative Evaluation of Near Regular Texture Synthesis Algorithms
Steve Lin,
James Hays, Chenyu Wu,
Vivek Kwatra,
and Yanxi Liu
IEEE Conference on Computer Vision and Pattern Recognition (CVPR) 2006
Paper, Bibtex
Quantitative evaluation is difficult for texture synthesis. Ground truth is not well defined.
But for certain textures you can objectively decide whether an algorithm has failed or not.
Regular and near-regular textures imply a definite structure that should be preserved.
We tested several popular algorithms on a large group of structured textures.
In addition to the CVPR 2006 paper, a more detailed technical report is available.
|
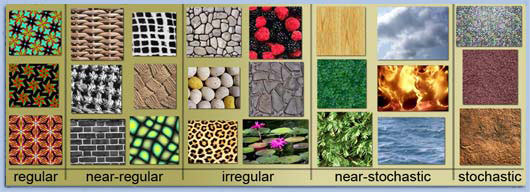
Near-Regular Texture Database - link
Online Database
We created a database of regular and near-regular textures for other researchers to use. You can submit your own textures, as well, and help the database grow.
|
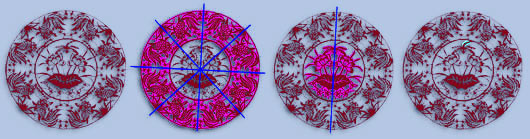
Digital Papercutting
Yanxi Liu, James Hays,
Ying-Qing Xu,
and Harry Shum
SIGGRAPH 2005 Sketch
Sketch, Bibtex
Papercutting is a widespread and ancient artform which, as far as we could tell, had no previous computational treatment. We developed algorithms to analyze the symmetry of papercut patterns and produce efficient folding and cutting plans.
|
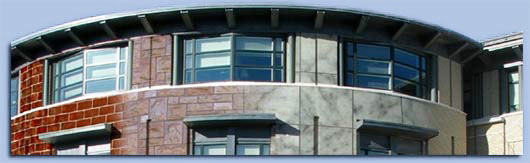
Near-Regular Texture Analysis and Manipulation
Yanxi Liu,
Steve Lin, and James Hays.
SIGGRAPH 2004
Project page, Paper, Bibtex
Abstract: A near-regular texture deviates geometrically and photometrically from a regular congruent tiling. Although near-regular textures are ubiquitous in the man-made and natural world, they present computational challenges for state of the art texture analysis and synthesis algorithms. Using regular tiling as our anchor point, and with user-assisted lattice extraction, we can explicitly model the deformation of a near-regular texture with respect to geometry, lighting and color. We treat a deformation field both as a function that acts on a texture and as a texture that is acted upon, and develop a multi-modal framework where each deformation field is subject to analysis, synthesis and manipulation. Using this formalization, we are able to construct simple parametric models to faithfully synthesize the appearance of a near-regular texture and purposefully control its regularity.
|
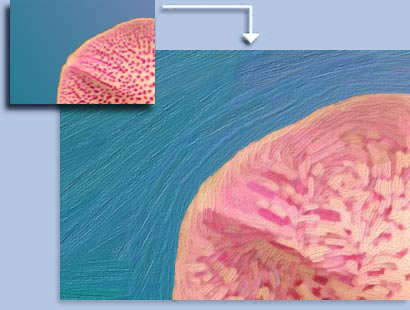
Image and Video Based Painterly Animation
James Hays and Irfan Essa.
NPAR 2004.
Project Page, Paper, Bibtex
We extend previous non-photorealistic rendering work to handle video significantly better by temporally constraining brush stroke properties in addition to other improvements.
|