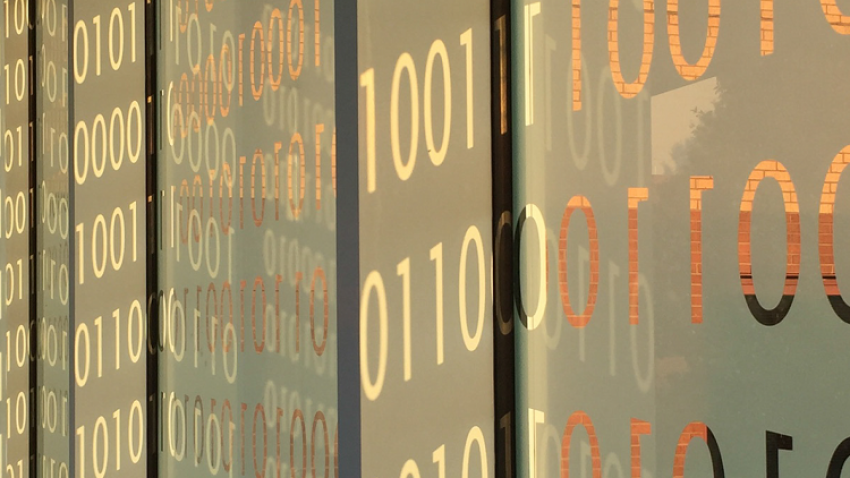
Bo Knows Neural Network Training – Xie in the Spotlight at Machine Learning Workshop
Training neural networks is no easy feat. Just ask Georgia Tech School of Computational Science and Engineering Ph.D. student Bo Xie.
However, in a presentation this week at the Simons Institute for the Theory of Computing at the University of California, Berkeley, Xie suggested a simple approach might be the most effective.
Xie was a guest speaker for a machine learning workshop and presented the event’s Spotlight Talk, titled Semi-Random Units for Learning Neural Networks with Guarantees.
During the talk, Xie described that despite the challenges of solving non-convex optimization problems, there is evidence that simple gradient-based algorithms may be effective in working toward minimizing neural network training errors.
Although these algorithms are widely used in practice, Xie – who expects to graduate this summer – said the jury is still out as to why they work so well in neural network training.
“It is a mystery, in theory, why it would work so well because training a neural network is a difficult, non-convex problem,” said Xie. “This means that gradient descent can easily get stuck in a bad local optimum.”
Bad local optima usually equate to a failure of learning for a neural network. With this failure, the only option is to start over again – possibly an exponential number of times – to achieve a global optimum, which is best described as a definitive best solution.
However, Xie’s research demonstrates that these solutions can be guaranteed with high probability using gradient-based algorithms. In turn, these positive outcomes represent successful learning for a neural network.
“In the short term, this work provides more understanding of the optimization learning landscape for a deep neural network,” said Xie. “We know more about why simple gradient descents will not be stuck in local optimal.
“Beyond this, my hope is that this work will inspire people to design more efficient algorithms for learning neural networks. It will allow us to train a better model with less time.”
Xie first became interested in machine learning as an undergraduate student at Beijing University of Posts and Telecommunications. He was intrigued by some early machine learning related technologies like face detection and spam email detection.
“I was fascinated about how to design algorithms that can learn from data instead of being manually programmed to do intelligent tasks,” said Xie.
Following his graduation, Xie plans to be a machine learning researcher in industry.
“I want to work on real-world large-scale problems. It is a really exciting time to do research in machine learning and artificial intelligence since they are transforming our lives in every aspect.”
Xie's primary academic adviser is Assistant Professor Le Song.
As computing revolutionizes research in science and engineering disciplines and drives industry innovation, Georgia Tech leads the way, ranking as a top-tier destination for undergraduate computer science (CS) education. Read more about the college's commitment:… https://t.co/9e5udNwuuD pic.twitter.com/MZ6KU9gpF3
— Georgia Tech Computing (@gtcomputing) September 24, 2024