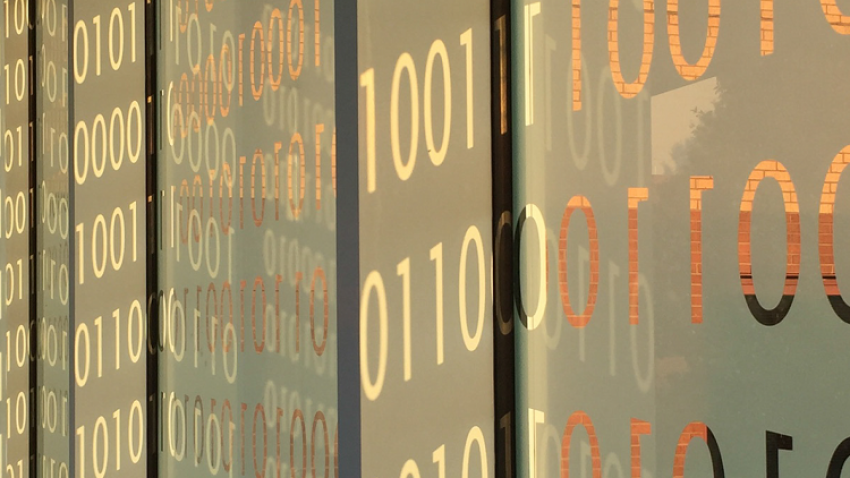
FairVis is Helping Data Scientists Discover Societal Biases in their Machine Learning Models
Researchers at Georgia Tech, Carnegie Mellon University, and University of Washington have developed a data visualization system that can help data scientists discover bias in machine learning algorithms.
FairVis, presented at IEEE Vis 2019 in Vancouver, is the first system to integrate a novel technique that allows users to audit the fairness of machine learning models by identifying and comparing different populations in their data sets.
According to School of Computational Science and Engineering (CSE) Professor and co-investigator Polo Chau, this feat has never been accomplished by any platform before, and is a major contribution of FairVis to the data science and machine learning communities.
“Computers are never going to be perfect. So, the question is how to help people prioritize where to look in their data, and then, in a scalable way, enable them to compare these areas to other similar or dissimilar groups in the data. By enabling comparison of groups in a data set, FairVis allows data to become very scannable,” he said.
In order to do accomplish this, FairVis uses two novel techniques to find subgroups that are statistically similar.
The first technique groups similar items together in the training data set, calculates various performance metrics like accuracy, and then shows users which groups of people the algorithm may be biased against. The second technique uses statistical divergence to measure the distance between subgroups to allow users to compare similar groups and find larger patterns of bias.
These outputs are then viewed and analyzed through FairVis’ visual analytics system, which is designed to specifically discover and show intersectional bias.
Intersectional bias, or bias that is found when looking at populations defined by multiple features, is a mounting challenge for scientists to tackle in an increasingly diverse world.
“While a machine learning algorithm may work very well in general, there may be certain groups for which it fails. For example, various face detection algorithms were found to be 30 percent less accurate for darker skinned women than for lighter skinned men. When you look at more specific groups of sex, race, nationality, and more, there can be hundreds or thousands of groups to audit,” said Carnegie Mellon University Ph.D. student Alex Cabrera.
Cabrera is the primary investigator of FairVis and has been pursuing this problem since he was an undergraduate student at Georgia Tech.
“During the summer of my junior year I had been researching various topics in machine learning, and discovered some recent work showing how machine learning models can encode and worsen societal biases. I quickly realized that not only was this a significant issue, with examples of biased algorithms in everything from hiring systems to self-driving cars, but that my own work during my internship had the possibility to be biased against lower socioeconomic groups.”
This is when Cabrera reached out to Chau who then recruited the help of CSE alumni Minsuk Kahng, CSE Ph.D. Fred Hohman, College of Computing undergraduate student Will Epperson, and University of Washington Assistant Professor Jamie Morgenstern.
Morgenstern is the lead researcher for a number of projects related to fairness in machine learning, including the study Cabrera mentioned about self-driving cars. This particular study shows the potentially fatal consequences of algorithmic bias which highlights the severity of software created without fairness embedded into its core.
FairVis is one of the first systems that helps us achieve a dramatic step towards understanding and addressing the problem of fairness in machine learning, and prevents similar headlines from making their way to reality in the future.
However, Cabrera stressed that the solution does not simply end with better data practices.
“Fairness is an extremely difficult problem, a so-called ‘wicked problem’, that will not be solved by technology alone,” he said.
“Social scientists, policy makers, and engineers need to work together to make inroads and ensure that our algorithms are equitable for all people. We hope FairVis is a step in this direction and helps people start the conversation about how to tackle and address these issues.”
As computing revolutionizes research in science and engineering disciplines and drives industry innovation, Georgia Tech leads the way, ranking as a top-tier destination for undergraduate computer science (CS) education. Read more about the college's commitment:… https://t.co/9e5udNwuuD pic.twitter.com/MZ6KU9gpF3
— Georgia Tech Computing (@gtcomputing) September 24, 2024