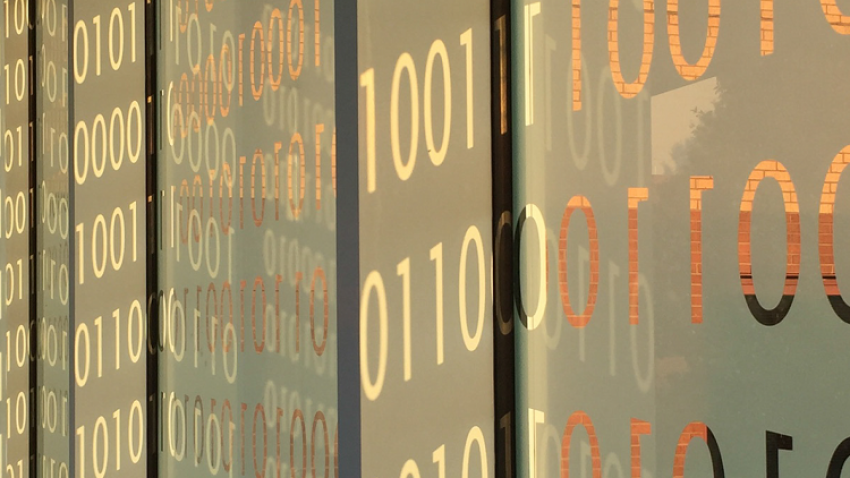
Meet CSE: Ph.D. Student David Betancourt is Designing AI to Help Us Monitor the Real and Surreal World
The School of Computational Science and Engineering (CSE) offers a uniquely interdisciplinary pool of student researchers who specialize in bridging software and hardware together with real-world applications ranging from bioinformatics to cybersecurity and more.
Today, we’d like to introduce you to David Betancourt, a CSE Ph.D. student and machine learning scientist with a passion for developing methods for artificial intelligence (AI) to act in real-life safety-critical situations.
Betancourt’s research has applications in a wide range of areas including physical infrastructure systems, finance, cloud computing, and cybersecurity. When he is not working on his Ph.D. thesis or appreciating surrealist art, Betancourt serves as the chief scientist at Vorstella, a tech startup using AI to stabilize and optimize cloud infrastructure systems.
Research Focus Areas: Machine Learning, Reinforcement Learning; Uncertainty Modeling; Computational Mechanics; Autonomous Decision-Making; Anomaly Detection; Time Series Prediction
Advisor: Rafi L. Muhanna, School of Civil and Environmental Engineering, Center for Reliable Engineering Computing
Hometown: Medellín, Colombia
Undergraduate Degree: Solid Mechanics
Current Program: Ph.D., Computational Science and Engineering
Tell us about your research interests.
I work in machine learning, uncertainty modeling, and numerical methods. The main goal of my research is to develop artificial intelligence that can reason and adapt under uncertainty in order to make AI safer.
How is uncertainty modeling research applied in the real world?
In most real-world applications, you have streams of data that contain significant uncertainty. One of the main problems in machine learning is that most times the data is far from being ready for learning---the data is unstructured, unlabeled, a large amount is missing, and exposed to uncertainty for multiple sources. In machine learning, we are used to data cleaning and data wrangling but less used to modeling the uncertainty in the data and in the models, especially if the source of uncertainty is more than randomness. As AI and machine learning make their way to more safety-critical real-world applications, obtaining reliable predictions is a necessity. Uncertainty modeling for machine learning seeks to make systems depending on AI safer, less risky, less biased, and more reliable.
It sounds like uncertainty modeling may help us more than we are aware of. Can you give us an example of how this is important and how your research is applied in this area?
Sure. For example, infrastructure systems, both physical and virtual, are essential to our society. One of the things that we are working on in our lab is connecting my research in machine learning and uncertainty modeling with structural health monitoring of public infrastructure to assess the real-time integrity of, for example, important bridges and tunnels.
With AI hardware and our algorithms in place, we can enhance the ability for monitoring systems to detect anomalies in real-time which alerts the engineers and other decision-makers in charge of a given infrastructure system that there is something wrong with it.
With more than 47,000 bridges in the United States being currently structurally deficient, your work may impact the entire country in a very tangible way. What type of hardware is your research using to help notify us of these bridges in need of repair?
That is indeed a huge concern. In that case, the main hardware consists of MEMS accelerometers which measure the forced and free vibrations of the system along with complementary environmental sensors that measure temperature, humidity, wind speed, etc. They are placed on different parts of the bridge system and are then used to detect and identify various types of damage [by measuring acceleration forces]. Although the basic sensors have been around for years most of the detection has unfortunately been post-hoc or even forensic by using multiple models of the systems. Instead, my research focuses on using machine learning to make anomaly detections in real-time using solely sensor data, while including uncertainty in the inputs and predictions. This can save lives and billions of tax-payer dollars.
Actually, this area is broadly known as activity recognition. For example, these sensors are very similar to the wearable accelerometers that are used to monitor Parkinson’s disease! In the case of Parkinson’s, you can detect activity that is anomalous and then based on that activity, you can customize the patient’s medical treatment. This is why interdisciplinary research is so important.
Can you tell us about the startup you are currently working in and what your role is there?
I am the chief scientist at a startup called Vorstella. We concentrate on AI monitoring for distributed systems on the cloud and we are developing machine learning algorithms to detect anomalies and optimize the cloud infrastructure of large companies. Without an AI detection system like ours, when the cloud systems fail, it can be very chaotic for the IT team to bring the system back up in a short amount of time. This is especially true if we’re talking about a hospital or a large payment network. As complexity in cloud computing infrastructure increases, it becomes necessary to bring AI to help you.
Why did you choose to come to Georgia Tech?
I’ve been Georgia Tech all the way! I came here for my undergrad and after getting my master’s in engineering I took a break for a few years to work as an R&D engineer and entrepreneur and then came back for my Ph.D. in CSE. I chose to come back to Georgia Tech because of the interdisciplinary collaboration between computing, engineering, and math. I checked multiple departments in the U.S. and CSE is the best PhD program when it comes to that collaboration.
Last, but certainly not least. What is an interesting fact about yourself?
I like to research the lives and motivations of a few artists and literary movements, kind of like a historian. Salvador Dalí and Leonard Cohen are at the top of my list. Depending on whom, I like to read their books, see their art in person, listen to their music, collect vinyl records, and visit their old homes and museums. There is a strong connection that you can make with people who enjoy the same artist and I’m fortunate to have friends who do.
As computing revolutionizes research in science and engineering disciplines and drives industry innovation, Georgia Tech leads the way, ranking as a top-tier destination for undergraduate computer science (CS) education. Read more about the college's commitment:… https://t.co/9e5udNwuuD pic.twitter.com/MZ6KU9gpF3
— Georgia Tech Computing (@gtcomputing) September 24, 2024