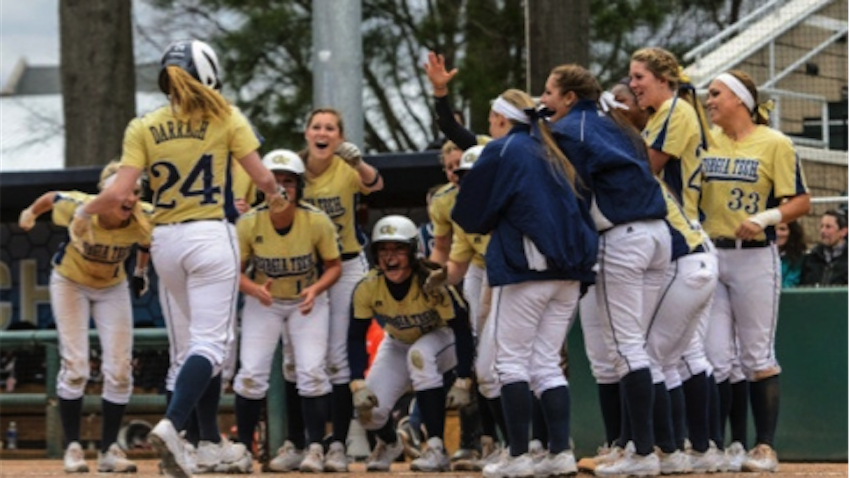
Pitch Perfect: GT Computing Undergrads Provide Automated Training Upgrade for Softball Team
There’s a classic story that former Atlanta Braves pitching coach Leo Mazzone used to share about Hall-of-Famer Greg Maddux, one of the smartest hurlers of all time. Although the exact details have changed in retelling over time, it goes something like this:
Maddux, a meticulous documenter of pitch sequences and batter results throughout his career, once explained to Mazzone in between innings that the leadoff batter in the following frame would pop out to third base on the fourth pitch of the at-bat. He’d start him with a fastball, change speeds for strike two, waste a pitch outside, and then induce the popup on a one-ball, two-strike count. Sure enough, a few minutes later, Maddux did exactly as he’d said.
There are a couple of lessons here: One, Maddux was a wizard. Many pitchers over time have tried to replicate his impeccable approach to the game, but few have ever succeeded at that level; two, pitch sequence matters – perhaps more than how overpowering your fastball is or how sharp the break is on your curve.
Capitalizing on this intuition, a group of undergraduate students at Georgia Tech are working with the softball team to provide an automated upgrade to players’ training. Using the wealth of statistics kept by the team – pitch-by-pitch data for balls, strikes, types of pitches thrown, and results – they have trained an algorithm that can select the best pitch to throw in any given situation.
The tool is used by the coaches and pitchers for game planning purposes, generating daily reports after every game and practice to help inform coaches of trends in sequences and results.
“In baseball and softball nowadays, data analytics has become such an incredibly important part of the game,” said Jack Bennett, a third-year Industrial Engineering student. “Anything that can get them data to go into games more prepared. Technology is at the forefront of this.”
They began using the approach during the 2019 season. Bennett and partners Zach Panzarino (third-year Computer Science) and Ron Kushkuley (third-year Computer Engineering) had demonstrated a similar capability at last year’s Sports Innovation Hackathon using data for Atlanta Braves pitcher Mike Foltynewicz, finishing in third place. Doug Allvine, assistant athletics director for innovation at Georgia Tech, put the team in touch with softball coach Aileen Morales. Morales was interested, and the students were able to begin testing the approach.
It works like this: The softball team keeps track of its own data – not just player statistics, but pitch selections and results for every pitcher in every game throughout the season. That’s a lot of data and can offer a lot of information. What happened when Pitcher X threw a 3-2 changeup to a lefthanded batter?
But it goes a little deeper than that. Panzarino, Bennett, and Kushkuley found that the pitch sequence is what matters most. That follows the standard strategic thinking – a slider away can be more effective if set up by an inside fastball on the previous pitch, for example. What the algorithm does, however, is consider the order each pitch is thrown in the at-bat and provide a score for which pitch will be most effective based on past data.
“We leverage sequences, the count, outs, everything,” Panzarino said. “Looking at the current state and the previous pitches, it will score all the potential future routes a pitcher can choose. We give them reports before each game so that they can prepare, and then we look at success or failure after the game.”
After a test run a year ago, the students have honed the technology and are working with the team again this year. Qualitatively speaking, they said they noticed results throughout the year.
“When we first gave them our analysis, it would recommend certain stuff in certain situations,” Bennett said. “Maybe it would say a changeup should be thrown more in this situation. Then, when we’d get postgame data later, we’d see that more changeups were being thrown and were continuing to be effective.”
“When I first saw what they were developing, I was beyond impressed,” Morales said. “We are very meticulous with collecting data in our program and trying to find ways to learn more about what is and what is not working for our athletes. It’s remarkable to see how they can take the data we had and leverage it in a way that allowed us to finetune our training.”
Recently, at the 2020 Sports Innovation Hackathon, the group developed a similar solution for baseball. They received runner-up in the competition, and hope to connect further with the Georgia Tech baseball team in the future.
“Tons of theory has been written on how pitchers should approach sequencing in games, but this is a model that can show you the data about how well that works,” Panzarino said.
As computing revolutionizes research in science and engineering disciplines and drives industry innovation, Georgia Tech leads the way, ranking as a top-tier destination for undergraduate computer science (CS) education. Read more about the college's commitment:… https://t.co/9e5udNwuuD pic.twitter.com/MZ6KU9gpF3
— Georgia Tech Computing (@gtcomputing) September 24, 2024