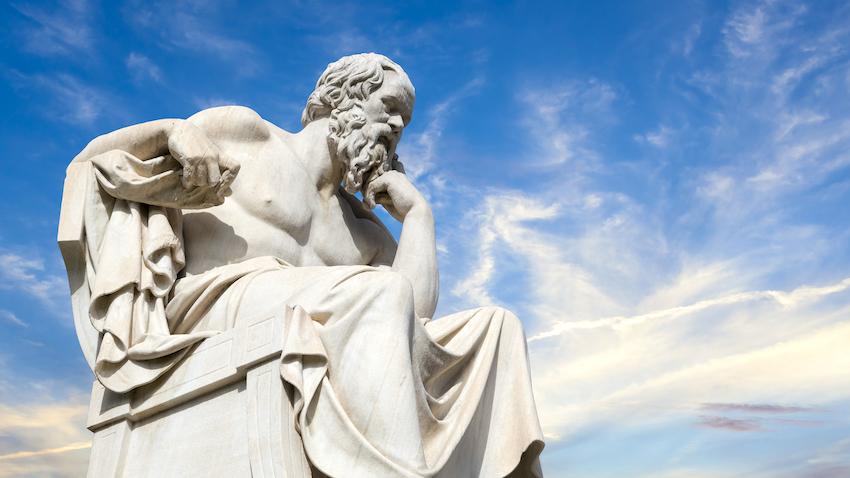
Embracing Socratic Paradox May Lead to More Reliable Predictions from AI Models
Researchers using new NSF and Facebook Research grants to better understand uncertainty
Next-generation artificial intelligence (AI) models used in public health, IoT, and other critical applications will soon be able to make better decisions and more accurate predictions thanks to a bit of philosophical wisdom being instilled in them at Georgia Tech.
School of Computational Science and Engineering (CSE) researchers are using a new 3-year, $1.1 million National Science Foundation (NSF) Medium grant to find ways of quantifying uncertainty in current AI models that use time-series data to make predictions.
From here, they hope to essentially teach the models what’s known as the Socratic paradox: “I know that I know nothing.”
“The key hurdle is that current deep learning neural models are poor at quantifying their uncertainty and are often overconfident in their predictions. Quantifying uncertainty will allow a model to say, ‘I don't know,’ when facing unknown or unexpected situations,” said B. Aditya Prakash, CSE associate professor and co-principal investigator for the project.
Because it doesn’t recognize what it doesn’t know, a current model may guess at answers and move forward as if it has guessed correctly. This is particularly problematic with time-series data – often used in public health monitoring and forecasting – where guesses and wrong answers can lead to lower levels of confidence in predictions generated by current-generation models.
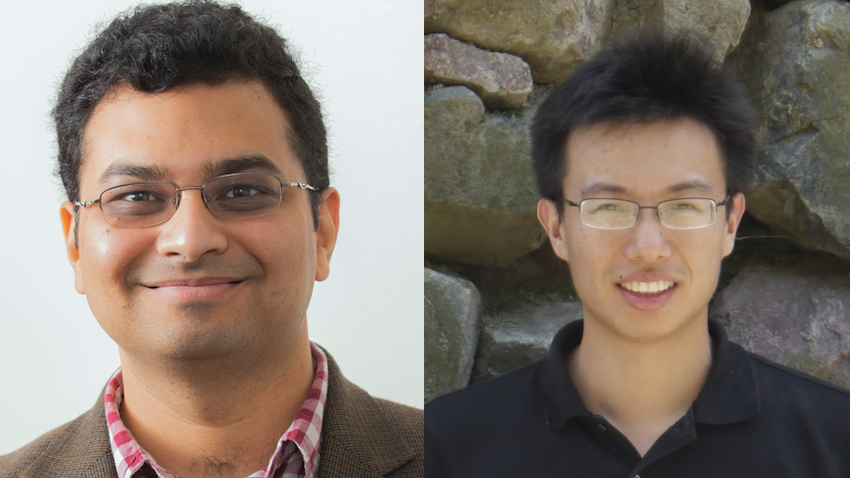
Prakash (left) and Chao Zhang (right), CSE assistant professor and lead PI, are working with co-PI Shuochao Yao from George Mason University to address these limitations. Along with quantifying the amount of uncertainty, the team is working to better understand the types and sources of predictive uncertainty.
“We need principled models which are flexible enough to model uncertainties from multiple sources in the datasets and also produce accurate predictions,” Zhang said. “Qualifying uncertainty will allow us to dynamically select a subset of models that are more reliable, which can improve the efficiency of the system and decisions at run time.”
Because so much depends on specific tasks and data, the researchers say it’s difficult to provide accurate estimates of efficiency improvements based on their novel approach. Preliminary results in disease forecasting, however, indicate the team’s new models, algorithms, and techniques can outperform previous state-of-the-art models up to 2.5x in accuracy and 2.4x in reliability.
The techniques and tools emerging from this project, formally titled Collaborative Research: Principled Uncertainty Quantification in Deep Learning Models for Time Series Analysis (NSF Award # 2106961), will be open source, and the research findings will be integrated into existing courses, tutorials, and workshops.
Facebook Research Award
Prakash and Zhang are taking another tack as well to improve the current state of predictive modeling.
The pair recently earned a Facebook 2021 Statistics for Improving Insights, Models, and Decisions research award. Their proposal, Non-Parametric Methods for Calibrated Hierarchical Time-series Forecasting, was one of 10 winners recently announced by Facebook Research.
According to the team, nonparametric statistical methods can be very flexible and effective for modeling time-series data when there are many unknowns in a dataset. Generally speaking, this is because nonparametric tools analyze group medians rather than group means. As a result, scientists can better understand outliers and can use them to strengthen.
“Our goal is to develop principled end-to-end models that incorporate hierarchical constraints and behaviors. We will also incorporate signals from different views such as demographics signals, time-series signals, and mechanistic models, to let them mutually reinforce each other to make the models more accurate, reliable, and robust,” said Zhang.
Once the project is complete, the results of the research will be used to improve predictive modeling in healthcare, public health, and a variety of industrial applications.
“For example,” said Prakash, “Facebook can use this technique to forecast demands in their data centers in different geographic regions. This will give them lead time to make their infrastructure more robust and efficient.”
As computing revolutionizes research in science and engineering disciplines and drives industry innovation, Georgia Tech leads the way, ranking as a top-tier destination for undergraduate computer science (CS) education. Read more about the college's commitment:… https://t.co/9e5udNwuuD pic.twitter.com/MZ6KU9gpF3
— Georgia Tech Computing (@gtcomputing) September 24, 2024