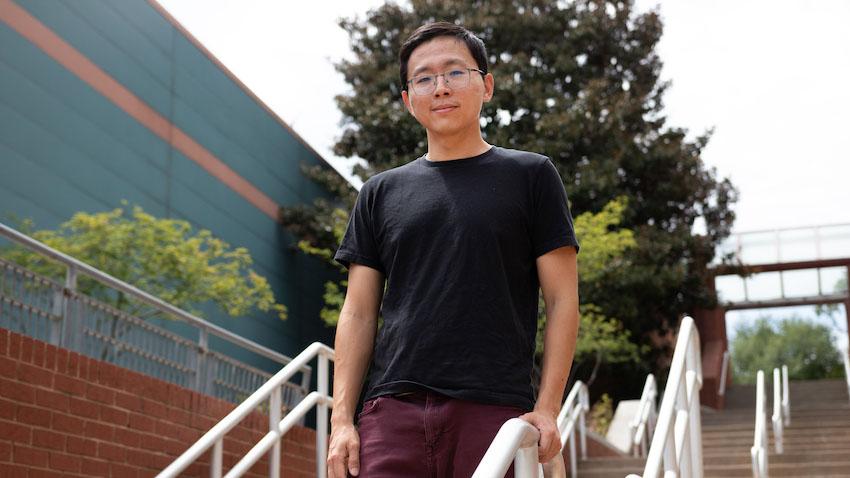
New Faculty Q&A with Danfei Xu
When it comes to robotics, Danfei Xu doesn’t consider himself a traditionalist.
His research focuses on data-driven approaches to develop better general-purpose robots that can work in everyday environments. While many robots are programmed through traditional analytical models that are based on equations, a data-driven approach allows robots to make decisions based on collected data.
Xu comes to the School of Interactive Computing after earning his Ph.D. in computer science from Stanford University in 2021. Xu will serve as an assistant professor. He is also a research scientist with NVIDIA AI Research. As an assistant professor at Georgia Tech, Xu will continue to build off his research and explore methods of learning and gaining intelligence autonomously within robotics and how humans can better teach robots.
In his first semester this fall, Xu will teach Deep Learning (CS 7643), which teaches complex, hierarchical feature representations from raw data and the fundamentals of principles, underlying mathematics, and implementation of details within.
Xu received his bachelor's degree in computer science from Columbia University and is originally from Shanghai, China.
What interests you about working at Georgia Tech?
The main reason is, if I want to spend a long time at a place, I want to be happy living here. I want to have a place where I can access a combination of big city and nature. There are not many places that has those things. I think Atlanta and Georgia Tech in general is a good choice. Georgia Tech is known for its robotics research, so that’s sort of a no-brainer.
What will your research consist of?
My research is in robots and specifically applying machine learning techniques to robotics problems. I think of that as a way for robots to learn by themselves and collect data by themselves. This is a slightly different approach to traditional robotics where they focus more on building a full analytical model of the world. We’re taking a more data-driven approach.
Within robotics, I mainly focus on manipulation problems. For example, we built an imitation-learning framework where we can allow humans to demonstrate tasks a few times or a few dozen times, depending on the difficulty of the task, and then collect these data. We then try to enable the robot to mimic the decision process, and then basically make that into a policy — a policy that controls the robot.
What inspired you to pursue this field of research?
I’ve always been interested in robotics, how to make things move like humans. I’m most interested in how the human brain works and how intelligence works in general. There are other ways to study this, but I thought it would be more fun to build something that can move around in the world and influence their environments through physical interaction.
What do you hope to accomplish in your research?
I want to continue to enhance my research with the support of students. The next milestone will be to build a comprehensive system that will allow us to sustain robotics research that allows us to show that a pure data-driven approach can enable robots to learn complex tasks. One of my goals is to learn for myself and disseminate what I know to my students on a different level.
What are you looking forward to about teaching your students and how do you plan on working with them?
The Deep Learning course teaches the basic principles of training large neural networks. I especially like the fact that the course gives the students hands-on experience with conducting basic research in AI and present the results to a wider audience. In terms of research, I enjoy mentoring and working alongside students to tackle impactful problems. My goal is to help them grow to become leaders in the community.
As computing revolutionizes research in science and engineering disciplines and drives industry innovation, Georgia Tech leads the way, ranking as a top-tier destination for undergraduate computer science (CS) education. Read more about the college's commitment:… https://t.co/9e5udNwuuD pic.twitter.com/MZ6KU9gpF3
— Georgia Tech Computing (@gtcomputing) September 24, 2024