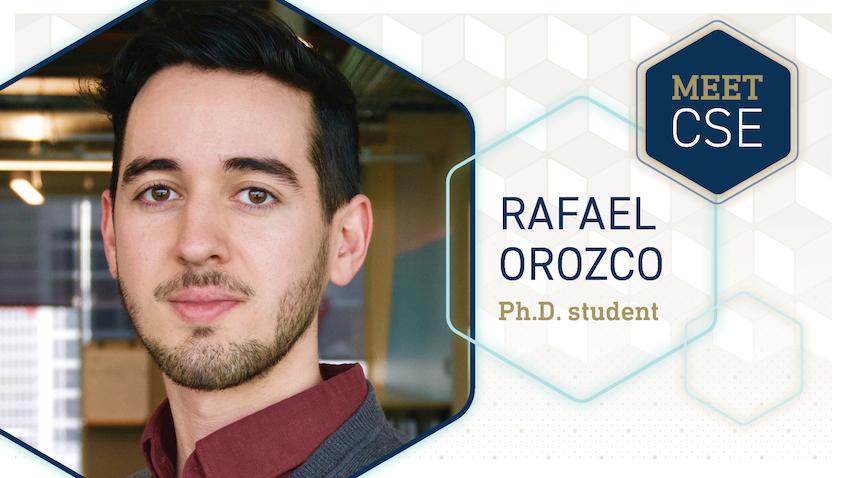
Meet CSE Profile: Ph.D. Student Rafael Orozco
The start of the fall semester can be busy for most Georgia Tech students, but this is especially true for Rafael Orozco. The Ph.D. student in Computational Science and Engineering (CSE) is part of a research group that presented at a major conference in August and is now preparing to host a research meeting in November.
We used the lull between events, research, and classes to meet with Orozco and learn more about his background and interests in this Meet CSE profile.
Student: Rafael Orozco
Research Interests: Medical Imaging; Seismic Imaging; Generative Models; Inverse Problems; Bayesian Inference; Uncertainty Quantification
Hometown: Sonora, Mexico
Tell us briefly about your educational background and how you came to Georgia Tech.
I studied in Mexico through high school. Then, I did my first two years of undergrad at the University of Arizona and transferred to Bucknell University. I was attracted to Georgia Tech’s CSE program because it is a unique combination of domain science and computer science. It feels like I am both a programmer and a scientist.
How did you first become interested in computer science and machine learning?
In high school, I saw a video demonstration of a genetic algorithm on the internet and became interested in the technology. My high school in Mexico did not have a computer science class, but a teacher mentored me and helped me compete at the Mexican Informatics Olympiad. When I started at Arizona, I researched the behavior of clouds from a Bayesian perspective. Since then, my research interests have always involved using Bayesian techniques to infer unknowns.
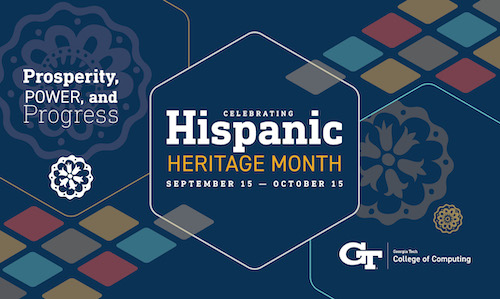
You mentioned your background a few times. Since it is National Hispanic Heritage Month, what does this observance mean to you?
I am quite proud to be a part of this group. In Mexico and the U.S., fellow Hispanics have supported me and my pursuits, so I know firsthand of their kindness and resourcefulness. I think that Hispanic people welcome others, celebrating the joy our culture brings, and they appreciate that our country uses the opportunity to reflect on Hispanic history.
You study in Professor Felix Herrmann’s Seismic Laboratory for Imaging and Modeling (SLIM) group. In your own words, what does this research group do?
We develop techniques and software for imaging Earth’s subsurface structures. These range from highly performant partial differential equation solvers to randomized numerical algebra to generative artificial intelligence (AI) models.
One of the driving goals of each software package we develop is that it needs to be scalable to real world applications. This entails imaging seismic areas that can be kilometers cubed in volume, represented typically by more than 100,000,000 simulation grid cells. In my medical applications, high-resolution images of human brains that can be resolved to less than half a millimeter.
The International Meeting for Applied Geoscience and Energy (IMAGE) is a recent conference where SLIM gave nine presentations. What research did you present here?
The challenge of applying machine learning to seismic imaging is that there are no examples of what the earth looks like. While making high quality reference images of human tissues for supervised machine learning is possible, no one can “cut open” the earth to understand exactly what it looks like.
To address this challenge, I presented an algorithm that combines generative AI with an unsupervised training objective. We essentially trick the generative model into outputting full earth models by making it blind to which part of the Earth we are asking for. This is like when you take an exam where only a few questions will be graded, but you don’t know which ones, so you answer all the questions just in case.
While seismic imaging is the basis of SLIM research, there are other applications for the group’s work. Can you discuss more about this?
The imaging techniques that the energy industry has been using for decades toward imaging Earth’s subsurface can be applied almost seamlessly to create medical images of human sub tissue.
Lately, we have been tackling the particularly difficult modality of using high frequency ultrasound to image through the human skull. In our recent paper, we are exploring a powerful combination between machine learning and physics-based methods that allows us to speed up imaging while adding uncertainty quantification.
We presented the work at this year’s MIDL conference (Medical Imaging with Deep Learning) in July. The medical community was excited with our preliminary results and gave me valuable feedback on how we can help bring this technique closer to clinical viability.
We are thrilled to announce Vivek Sarkar as the new Dean of the College of Computing at Georgia Tech! With a distinguished career spanning academia and industry, Sarkar's leadership promises to elevate our community to new heights. https://t.co/2mX5D46cJz pic.twitter.com/LxpLTCXWZV
— Georgia Tech Computing (@gtcomputing) April 12, 2024
@GeorgiaTech's dedication to excellence in computer science (CS) has been recognized once again, with the latest U.S. News and World Report rankings unveiling the institution at 7th place overall for graduate CS studies.https://t.co/qavNUSTb7n pic.twitter.com/BcGyGBQld8
— Georgia Tech Computing (@gtcomputing) April 10, 2024