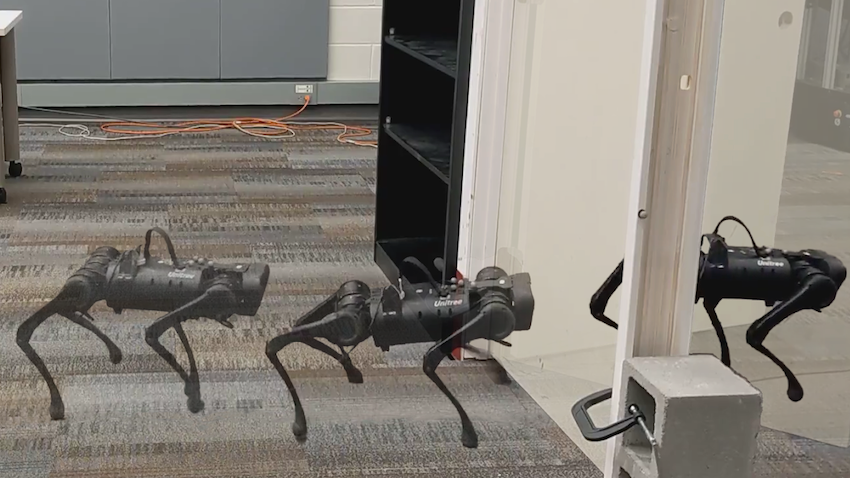
Novel Policy Allows Robots to Perform Interactive Tasks in Sequential Order
First, it walked. Then it learned to crawl under furniture. Next, it navigated to an object and pushed it across the floor.
And when the quadrupedal robot pushed open a door heavier than itself, a defining moment in the research of a Georgia Tech Ph.D. student arrived. The robot approached the door, stopped for a moment, bent its legs, and transferred that stored energy through a pushing motion to open it.
“I was relieved when that happened, and it was also exciting to see it work because this is something that hasn’t been done before,” said Niranjan Kumar. “We didn’t know if it was possible for the robot because it’s a small robot and the door is somewhat heavy.”
Thanks to Kumar’s new all-in-one residual learning policy, a quadrupedal robot can do an indefinite number of functions in a sequential order without having to relearn any motions.
It starts with learning a simple task while working its way through a set of tasks that become progressively more complex. Kumar calls this the Cascaded Compositional Residual Learning (CCRL) framework.
“Our approach is inspired by the way humans learn,” said Kumar, lead author of the recent paper Cascaded Compositional Residual Learning for Complex Interactive Behaviors. “Instead of directly learning a hard task such as interactive navigation, we start out with a simple skill.
“If you want to learn to play soccer, you don’t just go straight to soccer and teach yourself soccer. You first must learn to walk and run.”
Kumar’s paper was recently accepted to be published in Robotics and Automation Letters (RA-L), a journal publication of the Institute of Electricals and Electronics Engineers’ (IEEE) Robotics & Automation Society (RAS).
Typically, roboticists must train new control policies for a robot to perform each function, Kumar said. The training of individual skills often requires massive quantities of simulation samples to perform simple motor tasks through the time-consuming process of reward engineering.
The CCRL, however, functions as a “library” that allows the robot to remember everything it has learned while performing the simple tasks. Each newly obtained skill is added to the library and leveraged for more complex skills. A turning motion, for instance, can be learned on top of walking while serving as the basis for navigation skills.
Kumar said CCRL has broken new ground on interactive navigation research. Interactive navigation is one of several navigation solutions that allow robots to navigate in the real world. These solutions include point navigation, which trains a robot to reach a point on a map, and object navigation, which teaches it to reach a selected object.
Interactive navigation requires a robot to reach a goal location while interacting with obstacles on the way, which has proven to be the most difficult for robots to learn.
The key, Kumar said, to get a robot to go from walking to pushing an object is in the joints and the robot discovering the different types of motions it can make with them.
So far, Kumar’s policy has reached 10 skills that a robot can learn and deploy. The number of skills it can learn on one policy depends on the hardware the programmer is using.
“It just takes longer to train as you keep adding more skills because now the policy also has to figure out how to incorporate all these skills in different situations,” he said. “But theoretically, you can keep adding more skills indefinitely as long as you have a powerful enough computer to run the policies.”
Kumar said he sees CCRL being useful for home assistant robots, which are required to be agile and limber to navigate around a cluttered household. He also said it could possibly serve as a guide dog for the visually impaired.
“If you have obstacles in front of someone who is visually impaired, the robot can just clear up the obstacles as the person is walking, open the door for them, and things like that,” he said.
We are thrilled to announce Vivek Sarkar as the new Dean of the College of Computing at Georgia Tech! With a distinguished career spanning academia and industry, Sarkar's leadership promises to elevate our community to new heights. https://t.co/2mX5D46cJz pic.twitter.com/LxpLTCXWZV
— Georgia Tech Computing (@gtcomputing) April 12, 2024
@GeorgiaTech's dedication to excellence in computer science (CS) has been recognized once again, with the latest U.S. News and World Report rankings unveiling the institution at 7th place overall for graduate CS studies.https://t.co/qavNUSTb7n pic.twitter.com/BcGyGBQld8
— Georgia Tech Computing (@gtcomputing) April 10, 2024