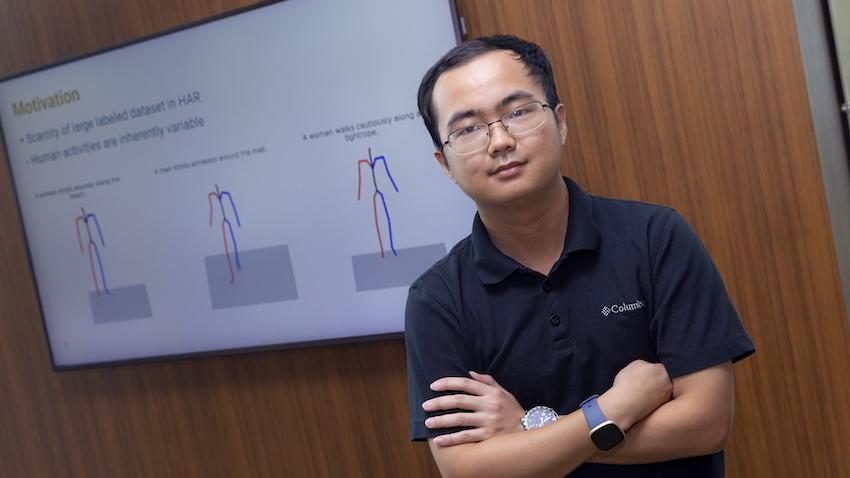
Student Shows ChatGPT Can Save Time, Resources for Sensory Data Researchers
A new data-gathering method could save sensory technology and human-activity recognition (HAR) researchers and data collectors a significant amount of time and money spent on resources.
In a recent paper, Georgia Tech third-year computer science major Zikang Leng introduced a large-language model approach with ChatGPT that could revolutionize how researchers collect sensory data.
Leng will present his paper this week at the 2023 Association of Computing Machinery’s joint conference on Pervasive and Ubiquitous Computing (UbiComp) in Cancun, Mexico. Generating Virtual On-body Accelerometer Data from Virtual Textual Descriptions for Human Activity Recognition is a best-paper nominee at the conference.
Data scientists and researchers gather sensory data for human-activity recognition to build wearable technology such as smartwatches and fitness trackers. Traditionally, this requires collecting hours of sensing data on human test subjects and then meticulously annotating that data on a massive scale.
“In the human activity recognition community, there’s the challenge of not having enough labeled data,” said Leng, who works in the Computational Behavior Analysis Lab directed by associate professor Thomas Ploetz. “To train a machine learning model that can recognize human activity based on sensory data, you need a lot of data. The labeling process is costly. You need to recruit and record human subjects, and you need a person to annotate the data. Thirty minutes of data could take 30 hours to annotate.”
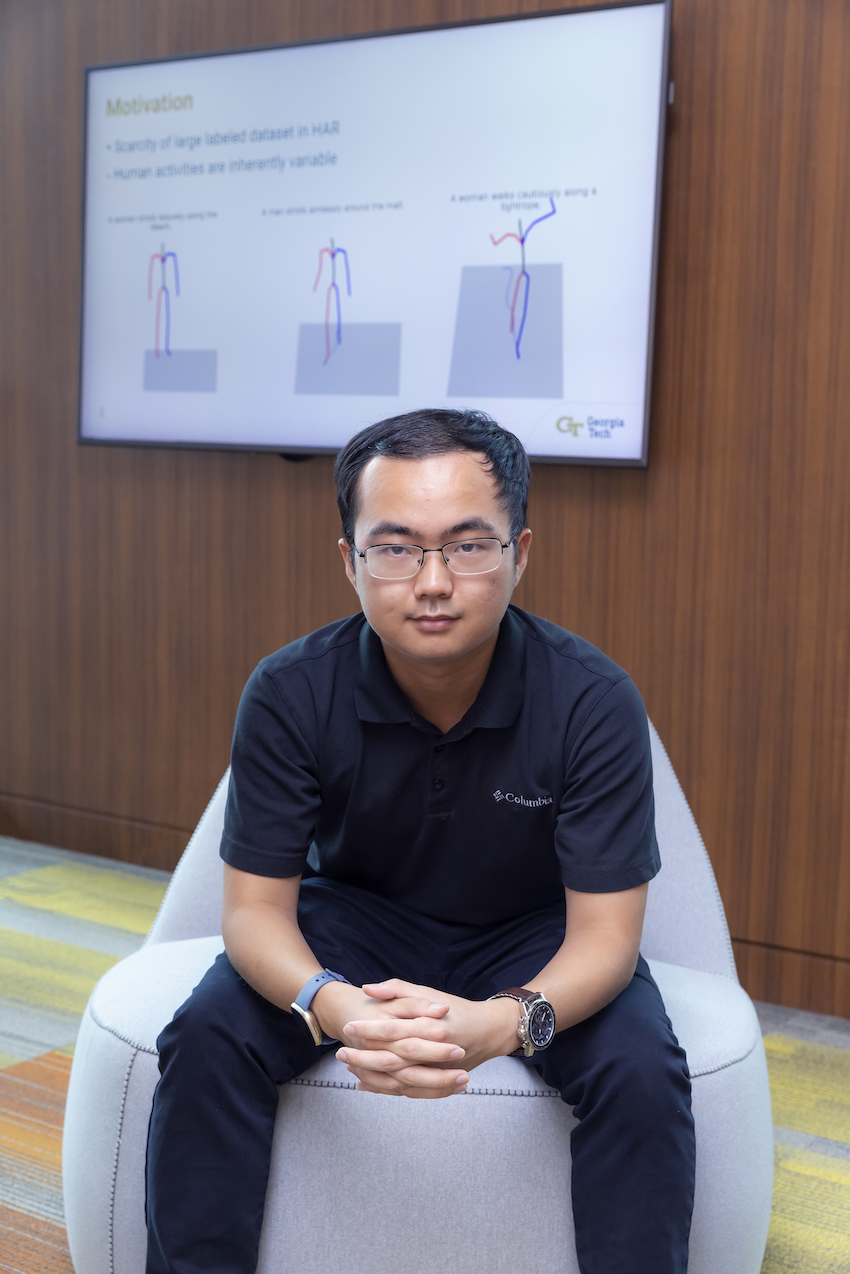
Leng said he initially set out to solve this problem by culling data from fitness videos on YouTube. However, after ChatGPT made its debut last year, he tried a new experiment. Leng prompted ChatGPT to provide hundreds of text descriptions of human motions such as walking, running, and jumping.
“Having diverse textual descriptions of how humans can perform certain activities is key,” Leng said. “Once we have a description of ‘a man jumping over a small gap and safely landing,’ we have a machine learning model that can convert the text into 3D animation. We analyze how the joints move from one frame to the next, and then we can extract the sensory data.
“That gives us hundreds of hours of sensory data for one activity. We’re creating the large-labeled dataset that we were lacking. We’re skipping the step of recruiting a human subject, and we’re skipping the annotation step. We’re saving loads of time and loads of resources.”
Leng tested his ChatGPT-based virtual dataset against three traditional datasets used within HAR research spaces. In the first two tests, the virtual data set scored slightly below its competitors while using a significantly lower quantity of data to train a model. For example, in one test, the virtual set used only 69 minutes of data to achieve a score similar to a real-world data set that needed 469 minutes to complete the training.
The virtual set scored higher in the third test. It require 41 minutes of data to train the model, while 1,107 minutes of the real-world set was needed. Leng said gathering 1,107 minutes of data in the real world would take months, but 41 minutes of data can be collected in real-time using ChatGPtT.
This novel method could have implications for small or startup tech companies that create wearable technology but don’t have the funds to hire human test subjects and data annotators.
“If you wanted sensing data for 50 activities, you’re looking at hundreds of human test subjects for a decent data set and you’re annotating that data frequently. That’s millions of dollars,” Zeng said. “If you’re just a startup, this is essentially free. The only thing that would cost money is a ChatGPT subscription.”
Leng said he recognizes there could be complications with ChatGPT’s propensity to display bias, but that shouldn’t taint his method because the activities he’s using to gather data are elementary and ubiquitous.
“ChatGPT is trained on the entire internet, and there is bias within the entire internet,” he said. “If it considers an activity specific to a minority, it might have a bad idea about how an activity can be performed. There may be a problem with that in the future, but we’re still at proof of concept, so we’re focusing on normal activities everyone can do.”
We are thrilled to announce Vivek Sarkar as the new Dean of the College of Computing at Georgia Tech! With a distinguished career spanning academia and industry, Sarkar's leadership promises to elevate our community to new heights. https://t.co/2mX5D46cJz pic.twitter.com/LxpLTCXWZV
— Georgia Tech Computing (@gtcomputing) April 12, 2024
@GeorgiaTech's dedication to excellence in computer science (CS) has been recognized once again, with the latest U.S. News and World Report rankings unveiling the institution at 7th place overall for graduate CS studies.https://t.co/qavNUSTb7n pic.twitter.com/BcGyGBQld8
— Georgia Tech Computing (@gtcomputing) April 10, 2024