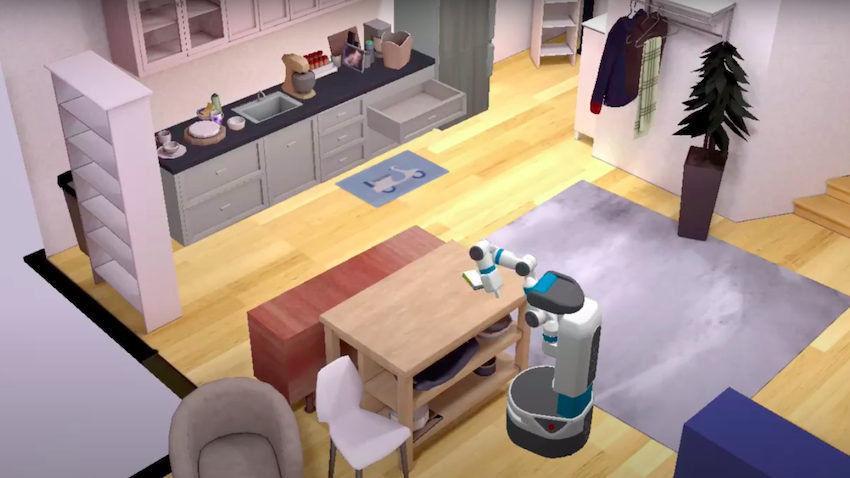
Exploring a Habitat: New Simulation Tool Helps Train AI to Perform Household Tasks
Household tasks like setting the table are so commonplace to humans that we often take for granted all the steps and decisions our brain makes throughout the entire process.
We open drawers and select the right utensils, pick them up, move to the table, and set each in a specific place around the surface. And what if a child has left their toy in front of somebody’s seat? Now that must be removed and returned to its proper location.
These complex, multi-step tasks are extremely challenging for current approaches in machine learning and robotics. A new paper presented by a 21-person team of researchers at last week’s NeurIPS conference aims to close this gap, offering improved interactive simulations of in-home tasks that will lay the groundwork for deployable home assistants in the future.
The work, colloquially referred to as Habitat 2.0, builds on Habitat, a three-dimensional simulation of a home environment in which an artificial agent gains vital information about its surroundings through interaction. The newest version of the simulation improves on the original by adding interactivity with physics simulations, a new dataset of interactive homes, and a new benchmark of home assistant tasks.
The improved physics of the environment allows simulation of a Fetch mobile robot picking up objects from clutter, opening drawers, closing the fridge, and more, improving upon simulation speeds and cutting down experimentation time. Things that would have taken up to six months previously can now be done in as little as two days.
“Habitat 2.0 will enable researchers to scale experiments to complex, realistic tasks in the home, like setting the table or cleaning up the kitchen,” said Andrew Szot, a Ph.D. student in Georgia Tech’s School of Interactive Computing and the first author on the paper.
“One reason tasks in the home are challenging is that in the home robots do not have information about the layout or where obstacles and objects are. Instead, they must extract this information from onboard sensors and egocentric visual perception.”
This is in addition to the complexity of multi-step tasks that require assessment and decision at every juncture. Now, with Habitat 2.0, simulations can more quickly train a robot to learn about its environment and potential obstacles to success, evaluate success or failure of the simulation, and retrain based on new parameters.
The platform, Szot said, lays the groundwork for achieving a research agenda in interactive embodied AI for years to come.
“Habitat 2.0 is a powerful tool for training and evaluating robot home assistants in simulation,” he said. “By training in simulation and then deploying to the real world, we hope that Habitat 2.0 helps progress toward household robot assistants that can help with everyday tasks.”
The paper is titled Habitat 2.0: Training Home Assistants to Rearrange their Habitat.
As computing revolutionizes research in science and engineering disciplines and drives industry innovation, Georgia Tech leads the way, ranking as a top-tier destination for undergraduate computer science (CS) education. Read more about the college's commitment:… https://t.co/9e5udNwuuD pic.twitter.com/MZ6KU9gpF3
— Georgia Tech Computing (@gtcomputing) September 24, 2024