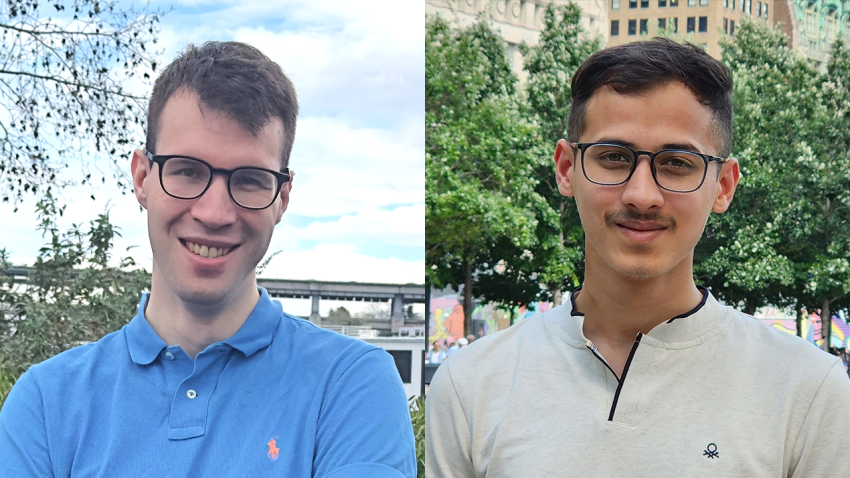
New Approach for Easily Merging Data Models Brings Adaptable, Multi-Tasking AIs Closer to Reality
Georgia Tech and IBM research ‘significantly enhances existing merging techniques for data tasks necessary to advance self-driving vehicles, chat assistants, and other AI applications
A new artificial intelligence innovation developed by Georgia Tech aims to improve the alignment of specialized data models by combining them to create a single, smarter AI that can perform many kinds of tasks well.
The new research tackles the challenge of effectively merging existing specialized models that are fine-tuned or optimized for a particular dataset using a broadly adopted fine-tuning method called LoRA. LoRA-based fine-tuning is attractive partly because it changes only a small part of the model, making it cost- and time-effective. For example, it can be applied to a dataset of cars to fine-tune it to recognize trucks.
“The challenge is that different LoRA fine-tuned models transform data in very conflicting ways, creating a key obstacle to effective merging,” said George Stoica, a Ph.D. student and co-lead investigator for the team. “To address this, we introduced KnOTS, a novel approach that explicitly aligns task-expert models without requiring data or training.”
The team’s results show that KnOTS boosts the performance of existing model-merging methods on datasets fine-tuned with LoRA by up to 4.3% when merging eight vision-based classification models used for images and video.
“KnOTS significantly enhances existing merging techniques, offering a plug-and-play solution for building smarter, more adaptable AI systems,” said Pratik Ramesh, Ph.D. student and the team’s other co-lead.
“A helpful analogy is to think about comparing and combining various color shades: it’s hard to directly compare them, but if you break each down into their red, green, and blue (RGB) components, they become much easier to compare, adjust, and blend.”
At its core, KnOTS makes comparing datasets easier and more accurate. It substantially reduces the conflicts between existing LoRA models and allows other merging algorithms to be applied more effectively.
[RELATED: Georgia Tech at ICLR 2025]
Merging data models allows for developing AI apps that can multi-task well. With current AI capabilities, Ramesh said it often feels like people are forced to juggle multiple apps or assistants.
“One helps you write, another helps with your taxes, and another translates languages,” he said.
But imagine having one AI that can do all of that effortlessly.
“Our method makes this future more realistic by taking a step toward the direction of enabling the fusion of specialized AIs into one super-assistant. This could mean faster, more versatile AI on your phone, smarter tools at work, or even more capable home assistants that understand and adapt to a wide range of needs—all without constantly switching between models.”
The team—from the School of Interactive Computing at Georgia Tech, along with a coauthor from IBM and MIT—also developed a new joint-task benchmark combining eight diverse datasets into a single unified task, where “KnOTS outperforms all baselines by up to 3.2%—demonstrating its ability to build truly general models.”
Stoica summarizes the team’s journey in tackling this broad challenge in the current AI landscape:
“From the inception of this project, it felt obvious to us that something was missing or an assumption was being violated when applying existing merging methods on LoRA models. Throughout our research, we were motivated to uncover overarching issues shared by these approaches.”
Stoica said it was very fulfilling to identify a solution that is ultimately both simple and lightweight.
The research paper, KnOTS: SVD Alignment for LoRA Model Merging, is part of the proceedings at the International Conference on Learning Representations, April 24-28, in Singapore. Georgia Tech team members include Stoica and Ramesh, computer science undergrad Boglarka Ecsedi, and Associate Professor Judy Hoffman. IBM and MIT Postdoctoral Researcher Leshem Choshen rounds out the team.
Learn more about Georgia Tech's research at ICLR 2025.
As computing revolutionizes research in science and engineering disciplines and drives industry innovation, Georgia Tech leads the way, ranking as a top-tier destination for undergraduate computer science (CS) education. Read more about the college's commitment:… https://t.co/9e5udNwuuD pic.twitter.com/MZ6KU9gpF3
— Georgia Tech Computing (@gtcomputing) September 24, 2024