Upcoming Events
CSE Faculty Candidate Seminar - Jacob Schreiber
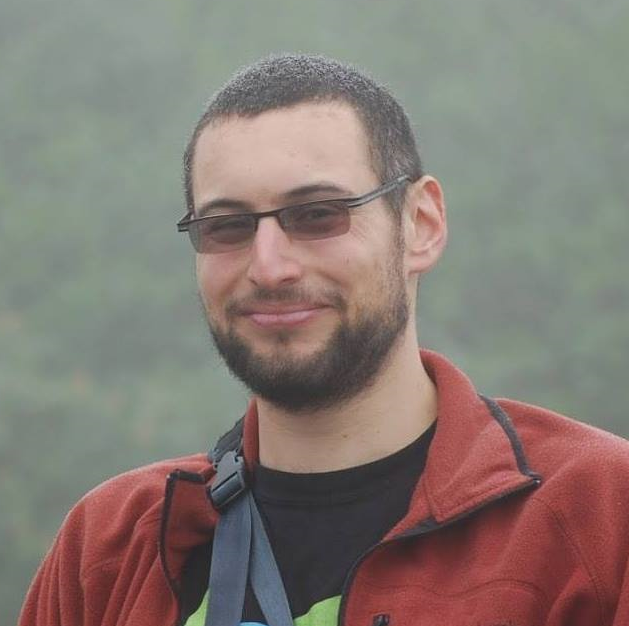
Name: Jacob Schreiber, postdoctoral scholar at Stanford University
Date: Thursday, February 29, 2024 at 11:00 am
Location: Coda Building, Second Floor, Room 230 (Google Maps link)
Link: The recording of this in-person seminar will be uploaded to CSE's MediaSpace
Coffee, drinks, and snacks provided!
Title: Dissecting the Cell Type-Specific Regulatory Role of Each Nucleotide in the Human Genome
Abstract: Proper regulation of gene expression is a crucial component of life, yet remains poorly understood despite a recent explosion in the quality and availability of genomic measurements. A paradigm that has emerged involves training neural networks that take in genomic sequences and predict these measurements directly. Far from being uninterpretable, these models can be paired with feature attribution algorithms to discover building blocks of the regulatory code. In this talk, I will introduce our ongoing work on a neural network called DragoNNFruit that extends this paradigm to modern data sets where measurements are available for each of many individual cells. A distinguishing feature of DragoNNFruit is that the parameters of this method are dynamically generated for each cell in the experiment based on properties of the cell, rephrasing the learning task as that of learning how to process genomic sequence in a cell-specific manner. When applied to data from cells that are slowly transitioning across types, DragoNNFruit uncovers the regulatory code of both endpoints but also how this code gets rewritten as cells alter their identity, and even how individual nucleotides can be involved in different regulatory programs in different cell types. Afterwards, I will briefly discuss pitfalls that one can encounter when applying machine learning to genomics data, and introduce my vision for new directions that these machine learning models can take.
Bio: Dr. Jacob Schreiber is a postdoctoral scholar in the Department of Genetics at Stanford University, where he develops machine learning-based methods for studying the genome. Previously, he did his Ph.D. at the University of Washington. In parallel to his research, he has contributed to the Python open-source ecosystem as a core developer for scikit-learn and the author of pomegranate, a package for probabilistic modeling, apricot, a package for submodular optimization, and ledidi, a method for designing biological sequence edits that exhibit desired characteristics, among others.
Event Details
Media Contact
Mary High
mhigh7@gatech.edu
EVENTS BY SCHOOL & CENTER
School of Computational Science and Engineering
School of Interactive Computing
School of Cybersecurity and Privacy
School of Computing Instruction
Algorithms and Randomness Center (ARC)
Center for 21st Century Universities (C21U)
Center for Deliberate Innovation (CDI)
Center for Experimental Research in Computer Systems (CERCS)
Center for Research into Novel Computing Hierarchies (CRNCH)
Constellations Center for Equity in Computing
Institute for People and Technology (IPAT)
Institute for Robotics and Intelligent Machines (IRIM)