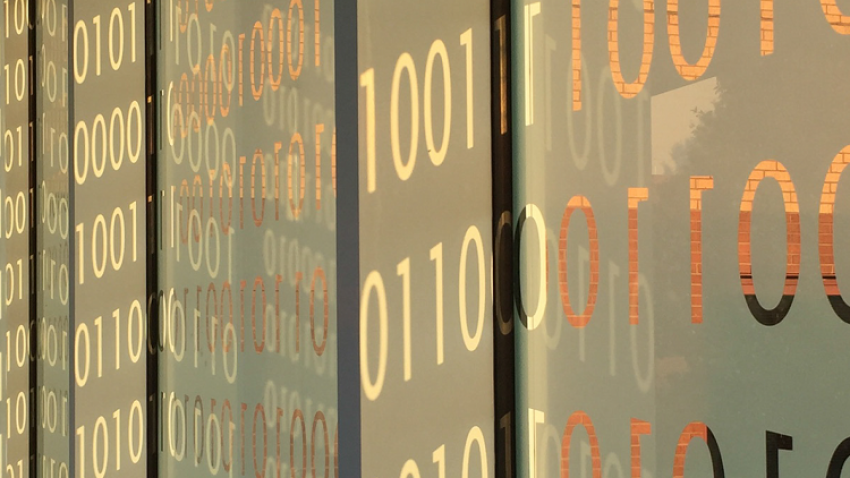
Jamie Morgenstern Wants to Bring Fairness to Machine Learning
Machine learning is used in almost every decision these days. It can help banks to find good lending candidates, employers to find qualified job applicants, and marketers to find to the right message. Local governments also rely on machine learning systems to allocate limited resources like police presence and social services.
Although automating these processes improves how quickly decisions are made, it can also exacerbate existing inequities. Machine learning models find patterns in historical data and use those to predict future patterns. If a certain population had more historical access to some resources (such as mortgages), a machine learning algorithm can identify this trend and try to replicate it in future decisions, thereby tilting things to that population's advantage.
This problem is something School of Computer Science Assistant Professor Jamie Morgenstern thinks about a lot. The entire machine learning pipeline needs to be scrutinized, from data gathering and cleaning to learning the model and deploying it.
Bridging the gap between economics and computer science
This application of game theory to economics has always interested Morgenstern, but it wasn’t until she took a computer science course in her sophomore year at the University of Chicago that she found the right outlet for it.
“I liked the implications of the theorems that showed how to solve problems that had some relation to the real world in computationally efficient ways,” she said.
One of these real-world problems is how to think about economics with limited data. Economists tend to believe the world has a perfect data distribution, and people behave rationally in their environments. Computer scientists, however, often operate with a worst-case scenario mindset and recognize people never have a perfect description of their environment — just historical data on it.
“Reasoning about strategic and worst-case behavior both is an interesting way to look at the world and find solutions,” Morgenstern said. “Neither is perfect, but both are useful models in different settings.”
For Morgenstern, machine learning is an ideal bridge between the two schools of thought because it allows generalizations about economic problems despite imperfect information. Morgenstern studied the social impact of machine learning, as well as the impact of social behavior on machine learning, during her Ph.D. at Carnegie Mellon University, where she was advised by renowned theorist Professor Avrim Blum.
Making machine learning fair
Fairness in machine learning is a relatively new area in an already burgeoning field, yet it’s important as the concept gains more popularity.
“The question is how should you make sure you aren’t over-tailoring the information you’re finding from this limited data while still learning?” Morgenstern said.
Morgenstern joined the SCS faculty in Spring 2018, choosing Georgia Tech for its reputation as a theoretical computer science hub ideal for the type of research she does.
[READ: Ethics Highlight 'Day of Machine Learning Discussion']
Her current work focuses on measuring how suboptimal data affects machine learning. A central goal of her work is to ensure machine learning makes the world a more equitable, ethical place rather than further entrenching historical injustices.
“I’ve always cared about social justice and the idea that we should make our systems more equitable and not profitable.”
As computing revolutionizes research in science and engineering disciplines and drives industry innovation, Georgia Tech leads the way, ranking as a top-tier destination for undergraduate computer science (CS) education. Read more about the college's commitment:… https://t.co/9e5udNwuuD pic.twitter.com/MZ6KU9gpF3
— Georgia Tech Computing (@gtcomputing) September 24, 2024