Upcoming Events
School of CSE Seminar Series: Mikio Aoi
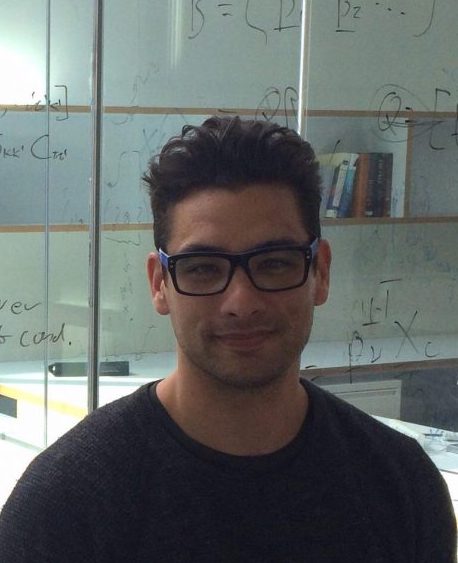
Speaker: Assistant Professor Mikio Aoi, University of California, San Diego
Date and Time: November 3, 2:00-3:00 p.m.
Location: Coda 230
Host: School of CSE Assistant Professor Anqi Wu
Title: Examining Shared Structure Across Multivariate Datasets
Abstract: A number of recent studies have sought to understand the behavior of artificial and biological neural networks by comparing the structure of representations across layers, networks and brain areas. Increasingly prevalent, too, are comparisons across modalities of data, such as neural network activations and training data or behavioral data and neurophysiological recordings. Many of these studies use dimensionality as a proxy for computational complexity, measured by approaches such as canonical correlation analysis (CCA), but a standard, model-free measure is lacking. We present a candidate measure for shared dimensionality that we call the effective number of shared dimensions (ENSD). We illustrate how the ENSD and its constituent statistics can be applied in a variety of analyses, including on across-layer similarities of neural network activations and communication subspaces between brain regions. By comparing it to existing metrics we find that the ENSD can be re-written as a scaled version of centered kernel alignment (CKA) that additionally describes the dimensionality of the aligned subspace and, unlike CCA, is robust to cases where data is sparse or low rank. We also include a novel analysis on the organization of the fly olfactory system, where the ENSD provides insight into the interaction between innate and learned olfactory representations. Altogether, we present an interpretable and computationally efficient model-free measure of shared dimensionality that can be used to probe shared structure in a wide variety of data types.
Bio: Dr. Aoi is an Assistant Professor of Data Science and Neurobiology at the University of California, San Diego. His work is aimed at the development of statistical and machine learning techniques for the analysis of neural population activity with the aim of determining the computational principles of biological neural networks.
Event Details
Media Contact
Host: Anqi Wu (anqiwu@gatech.edu)
EVENTS BY SCHOOL & CENTER
School of Computational Science and Engineering
School of Interactive Computing
School of Cybersecurity and Privacy
Algorithms and Randomness Center (ARC)
Center for 21st Century Universities (C21U)
Center for Deliberate Innovation (CDI)
Center for Experimental Research in Computer Systems (CERCS)
Center for Research into Novel Computing Hierarchies (CRNCH)
Constellations Center for Equity in Computing
Institute for People and Technology (IPAT)
Institute for Robotics and Intelligent Machines (IRIM)