Upcoming Events
CSE Faculty Candidate Seminar - Lu Mi
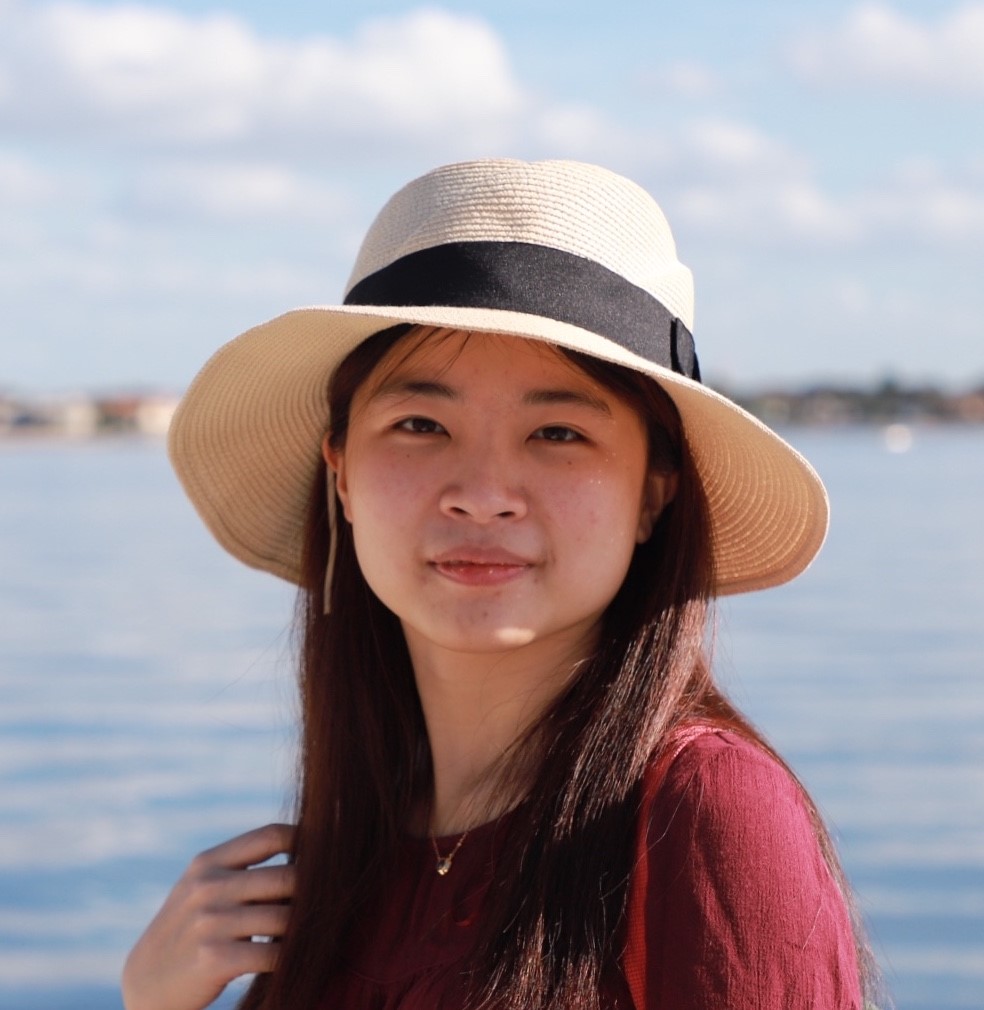
Name: Lu Mi, Shanahan Foundation Fellow at the Allen Institute for Brain Science, University of Washington
Date: Thursday, March 14, 2024 at 11:00 am
Location: Scheller College of Business Building, 1st Floor, Room 102 (Google Maps link)
Link: The recording of this in-person seminar will be uploaded to CSE's MediaSpace
Title: Accelerating Scientific Discovery in the Brain with Machine Learning
Abstract: The inner workings of the brain are complicate and mysterious, and understanding these mechanisms has significant implications for healthcare and artificial intelligence. Recent technological advancements have enabled the study of the brain at single-cell resolution, utilizing multi-modal neural data, including genomics, synaptic connectivity, neuronal activities, and animal behaviors. However, the mammalian brain’s vast scale, ranging from millions to billions of neurons, presents a challenge in developing generalizable and scalable solutions.
My research focuses on the AI-driven paradigm to overcome these challenges and accelerate biological and scientific discoveries in the brain. My contributions can be broadly classified into three main categories: (1) The use of advanced machine learning tools to create fast and scalable automatic imaging pipelines. This includes developing active acquisition pipelines to speed up electron microscope imaging data collection, and designing parallel, scalable algorithms for automatic reconstruction of nanoscale synaptic connectivity; (2) The development of generalizable and interpretable frameworks to understand brain function using multi-modal neural data. My work uses artificial neural networks as expressive solutions to predict complex neural dynamics, and learning representations that allow in-vivo identification of genetically identified cell types and connectivity. By integrating mechanistic models from neuroscience as biophysics priors, we could infer unmeasured information and allow testing various hypotheses; (3) The application of machine learning for guiding experimental designs. This involves using active learning to design optimal stimulation patterns that generates most informative data for system identifications.
Looking forward, my future work aims to develop foundation models in neuroscience for data-driven discovery. Meanwhile, extending from understanding brain functions, I am interested to explore healthcare applications and develop brain-inspired AI frameworks, and bridge the gap between artificial and biological neural networks.
Bio: Lu Mi is a Shanahan Foundation Fellow at the Allen Institute for Brain Science and the University of Washington where she collaborates with multiple investigators, including Dr. Uygar Sümbül, Prof. Matt Golub, Prof. Edgar Walker, and Prof. Eric Shea-Brown. She earned her Ph.D. degree in Computer Science from MIT in 2022, advised by Prof. Nir Shavit. Concurrently, she was a fellow in the Center for Brain Science at Harvard University from 2020 to 2022. She received a bachelor’s degree from Tsinghua University. Her research lies at the intersection of natural intelligence and artificial intelligence. She mainly focuses on building fast and scalable automatic brain imaging pipelines. And by leveraging artificial neural networks, she aims to understand the mechanisms of coding, computation, and learning within biological neural networks, using multi-modal neural data. Her research is recognized with several honors, including the MathWorks Fellowship, Shanahan Foundation Fellowship, and an NIH award. She was selected as Rising Stars in EECS in 2022 and her work has been featured in MIT News and Forbes.
Event Details
Media Contact
Mary High
mhigh7@gatech.edu
EVENTS BY SCHOOL & CENTER
School of Computational Science and Engineering
School of Interactive Computing
School of Cybersecurity and Privacy
School of Computing Instruction
Algorithms and Randomness Center (ARC)
Center for 21st Century Universities (C21U)
Center for Deliberate Innovation (CDI)
Center for Experimental Research in Computer Systems (CERCS)
Center for Research into Novel Computing Hierarchies (CRNCH)
Constellations Center for Equity in Computing
Institute for People and Technology (IPAT)
Institute for Robotics and Intelligent Machines (IRIM)